Brains in a Dish are the Future of Computing
Neurons playing video games, synthetic biological intelligence and the nano-intentionality of technology
I have no mouth and I must play Pong
Somewhere in a biotech laboratory in Australia, a small clump of human neurons is teeming with microscopic sparks of electricity. This mini-brain in a dish lacks any means of perception or action: all it knows about reality is delivered as electrical current signals from a small electrode array. Entirely cut off from the world, it attempts to make sense of its existence by searching for predictable patterns and adapting the structure of its neural network accordingly. It has but one purpose: to play the 1972 Atari videogame Pong.
In 2022, an article published in the biology journal “Neuron” announced the birth of DishBrain, an artificial intelligence system based on a culture of living human and rat neurons. The authors, founders of the biological computing startup Cortical Labs, demonstrated the cognitive abilities of DishBrain by successfully training the neurons to play a classic arcade video game, Pong. Not only is DishBrain capable of successfully learning to play Pong without any prior instruction on its rules, but according to the authors it exhibits signs of “sentience” when “embodied” within the game simulation. Although the choice of vocabulary makes the experiment sound like a dystopian nightmare, the technology—which the authors termed synthetic biological intelligence—is much more subtle than it might seem.
Neuron cultures can be grown from different sources. Often, they originate from induced pluripotent stem cells (iPSCs). They are regular, adult somatic cells “reprogrammed” by introducing specific genes into their DNA through a technologically altered virus (a process similar to the one used to deliver vaccines). iPSCs technology works like a “time machine”, returning adult cells to their embryonic, unspecialized state. From this state, under controlled physicochemical circumstances, the cells can be induced to differentiate once more, developing into neurons or any other specialized cell type. In the case of DishBrain, these neurons were grown over an array of eight electrodes designed to register spikes of electrical activity and provide feedback to the cells in the form of electrical current.
Let me briefly introduce the theoretical framework behind this experiment before illustrating how the system works. The training method of this biological neural network is based on a theory of cognition known as active inference, proposed by neuroscientist Karl J. Friston (also one of the DishBrain paper’s authors). Within the framework of this theory, intelligent behavior is understood as emerging from a simple principle, i.e., the minimization of surprise. According to the principle of active inference, all cognitive systems tend to reduce the likelihood of encountering unpredictable patterns of perception that might threaten the system’s integrity. In this context, words like “perception” and “surprise” should not be understood strictly in their anthropomorphic sense. The theory of active inference can be applied to any physical system affected by environmental changes and capable of responding to these changes with a degree of control.
In the context of DishBrain, the principle of active inference was materialized by exposing neurons to different electrical stimulation patterns. When the neurons in the dish failed to produce electrical activity that would direct the paddle onto the ball, they were subjected to chaotic and unpredictable electrical stimuli. In turn, they were rewarded with regular, predictable stimulation when they successfully deflected the ball. By using this closed-loop electrical stimulation system, neurons receive feedback on their decisions in real-time. According to the authors, this feedback mechanism results in an “embodiment”: it creates a meaningful connection between the simulated game environment and the biological neural network’s actions. Within a few hours of training, and without any other direction from the outside, DishBrain learned to modify its electrical activity to play the game successfully.
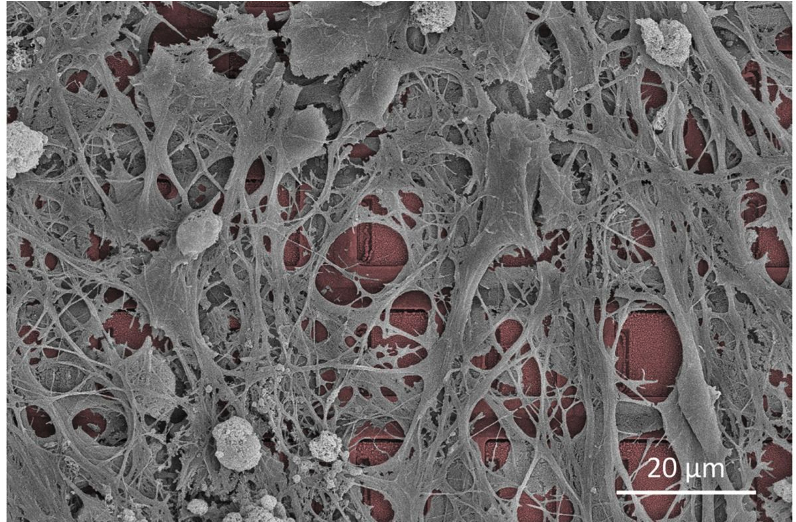
Synthetic biological intelligence
One of the reasons for the rising interest in biological computing today is its time and energy efficiency compared to conventional computing systems. The human brain is regarded as the most powerful supercomputer, capable of performing one quintillion (1018) floating point operations with only 20 watts of energy compared to the tens of megawatts required by silicon-based supercomputers. With almost 100 billion interconnected neurons, human brains can process and access information exponentially faster than any human-made technology. But the difference between biological and digital computing isn’t just a matter of energy or speed. What makes synthetic biological intelligence interesting isn’t just whether it can match or surpass the performance of silicon-based AI, or whether it can play arcade video games, but which different technological and cognitive possibilities it might disclose.
Some of the most fascinating findings of the DishBrain paper aren’t the most obvious. The study demonstrated the neurons' capacity to self-organize into distinct regions specialized at performing specific tasks, a trait known in neurobiology as “functional plasticity”. Much like it happens in the brains of living organisms, self-organization into a modular structure allows the synthetic-biological neural network to respond more effectively to uncertainty in the simulated environment. In addition, the authors remark on the difference in behavior between mouse and human neuron cultures. While mouse neurons are better at playing the game initially, human neurons appear capable of faster learning, seemingly engaging in more “explorative” in-game movements. It’s important to note how this difference has nothing to do with the system’s training but arises from the materiality of each specific neuronal tissue: it emerges from biology rather than programming. While these differences have limited consequences in the context of Pong, we can speculate on how they might affect more complex cognitive tasks. Wetware systems are unique in their histories and material makeup, resulting in different abilities and behaviors. Can we imagine a future where computers, like animals and people, each possess intrinsic capacities and predispositions?
Since the development of DishBrain in 2022, wetware systems have grown more complex. While DishBrain is a two-dimensional layer of cells, scientists are already working on wetware computing systems based on brain organoids—that is, three-dimensional miniature organs. This year, the first platform for organoid computing research, Neuroplatform, was developed by the Geneva-based research startup FinalSpark. Neuroplatform allows researchers worldwide to work on the biological chip remotely, testing different behaviors and training methods. Biological neural networks like Neuroplatform can also be trained with neurotransmitters: releasing dopamine into the system rewards the neurons and provides positive reinforcement for learning. As the technology for wetware computing develops, we can expect biological neural networks to engage in a much broader range of activities than just playing vintage arcade video games.
“Nano-intentionality” of technology
The development of biological computing raises new questions on artificial intelligence, and, more generally, on the possibility of developing intelligent and sentient technologies. According to neuroscientist W. Tecumseh Fitch, silicon-based AI is structurally incapable of truly intelligent behavior because of its “hard” material substrate. Fitch claims that conventional computer chips, composed of inorganic materials that don’t perceive or adapt to the world around them, will never match biological systems’ flexibility and behavioral complexity. He coined the term “nano-intentionality” to refer to the capacity of living structures (from simple amoebas to human brains) to perceive the world and adapt accordingly. “A crucial difference between a cell and a transistor on a silicon chip,” he argues, “is that the former arrangement of matter can autonomously and adaptively modify itself in response to its circumstances, whereas the latter cannot”.
If we subscribe to this theory, wetware computing might be the first step towards achieving a “nano-intentional” AI. By incorporating soft, adaptable living matter within a larger technological system, innovations like DishBrain might close the gap between synthetic and biological minds. Nano-intentionality is a powerful idea when applied to the future of technology. It suggests that designing artificial systems from materials capable of perception and adaptation is the key to understanding, and gradually bridging, the difference between biological and artificial cognition. Nano-intentional AI might be more than just a more energy-efficient twin of the silicon-based AI we already have: it might embody an entirely new, unprecedented form of technological intelligence.
In his book Ways of Being, James Bridle proposes three guiding principles for the future of computing that might help us build ecological and less exploitative technologies. “Our machines”, writes Bridle, “should be non-binary, decentralized and unknowing”. In his words, “to exist in a state of unknowing demands a kind of trust in ourselves and in the world to be able to function in a complex, ever-shifting landscape over which we do not, and cannot, have control.” What I find most interesting about the DishBrain case study is how the neurons’ behavior, while directed towards a goal, isn’t entirely predictable. Under the same conditions, each cell culture finds unique and unforeseen paths toward solving a given problem. The same training protocol might lead to different solutions, emerging from each neuron’s phylogenetic and ontogenetic history. When considering traditional computing applications, this element of “unknowability” in our relationship with technology can, in comparison to “hard”, deterministic systems, be regarded as a flaw. However, if our approach towards technology becomes softer, it might reveal itself as the root of new technological creativity and inventiveness. Perhaps neurons in a dish will never be very good at playing Pong, but—if we let them—they might be able to do much more surprising things.