2.2 Million New Materials Discovered with AI
Geological media, the technosphere, and the impending singularity
In my days as a young chemistry student, now long gone, I learned that there were two ways of being a chemist. Some of us were destined to embody the archetype of the lab rat, wearing a white coat while working with fuming mixtures of dangerous chemicals. Others would commit themselves to a more obscure practice. Brewing languages and numbers instead of fluids and crystals, these other chemists’ labs were not rooms full of glassware and reagents, but terminals connected to supercomputers.
These two paths were self-exclusionary: devoting oneself to one meant (in the minds of overly zealous twenty-year-old aspiring scientists) being certain of the inadequacy of the other. While experimental chemists were convinced that only they could master the true nature of our ancient science, through fearless proximity to the explosive energy of untamed matter, computational chemists regarded the knowledge of their colleagues as little more than pre-modern fluff. To them, cracking the complexity of chemistry was only a matter of combining quantum physics with enough computational power.
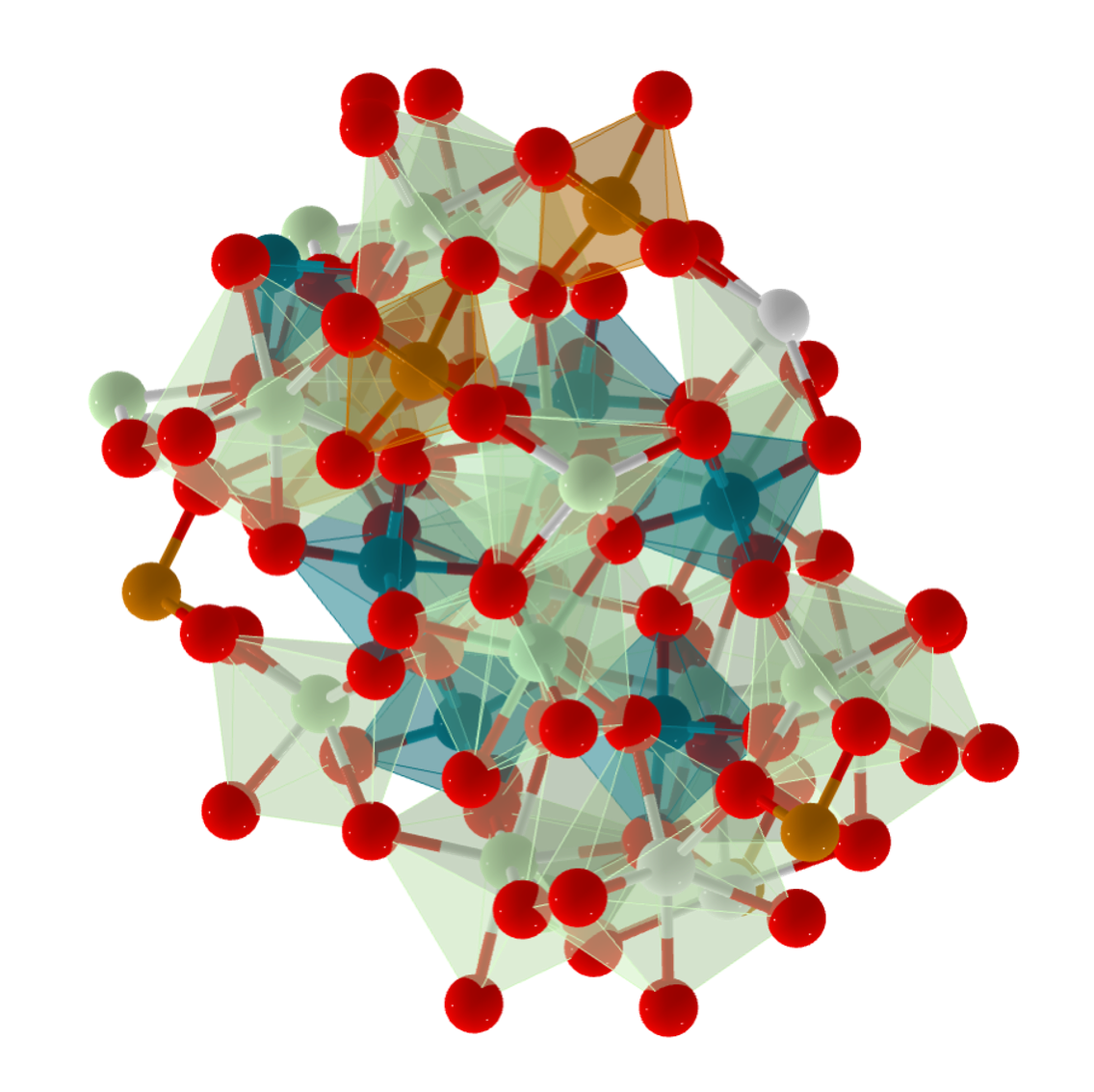
At the time, something about the possibility of turning chemistry into a “hard science” was alluring to me. This was partly due to my naive fascination with huge and scary formulas; it is likely that my natural incompetence in organic chemical synthesis (and the stinging resentment that ensued) also played a role. But there was also a deeper curiosity. To test the limits of mathematical language and computational infrastructure in their ability to explain, represent, and possibly even create material reality.
Metamateriality
My younger self would have been thrilled to learn that last November the world of materials science was shaken by a groundbreaking announcement. Google’s AI, DeepMind, discovered 2.2 million new inorganic materials unknown to humanity, suddenly expanding the 40,000 materials previously known to our species by two orders of magnitude. This unprecedented discovery is the work of a technological platform called Graph Networks for Materials Exploration, a.k.a. GNoME; the resulting materials can be explored freely in an open-source online database. But what does it even mean for an AI to “discover” a material? What does a disembodied algorithm have to do with something as tangible as any garden rock?
To answer this deceivingly straightforward question, you need some context first. You should know that the rules determining how elements combine in real-world materials aren’t as obvious as you might think. Materials chemistry is like learning English as a second language: they teach you the rules in school, but you soon learn that most of them rarely apply to the words you encounter in the wild. Atoms, like words, aren’t objects like any other. Although we represent them as little hard and perfect spheres, atoms are complex, non-classical bodies that interact with each other in counterintuitive ways.
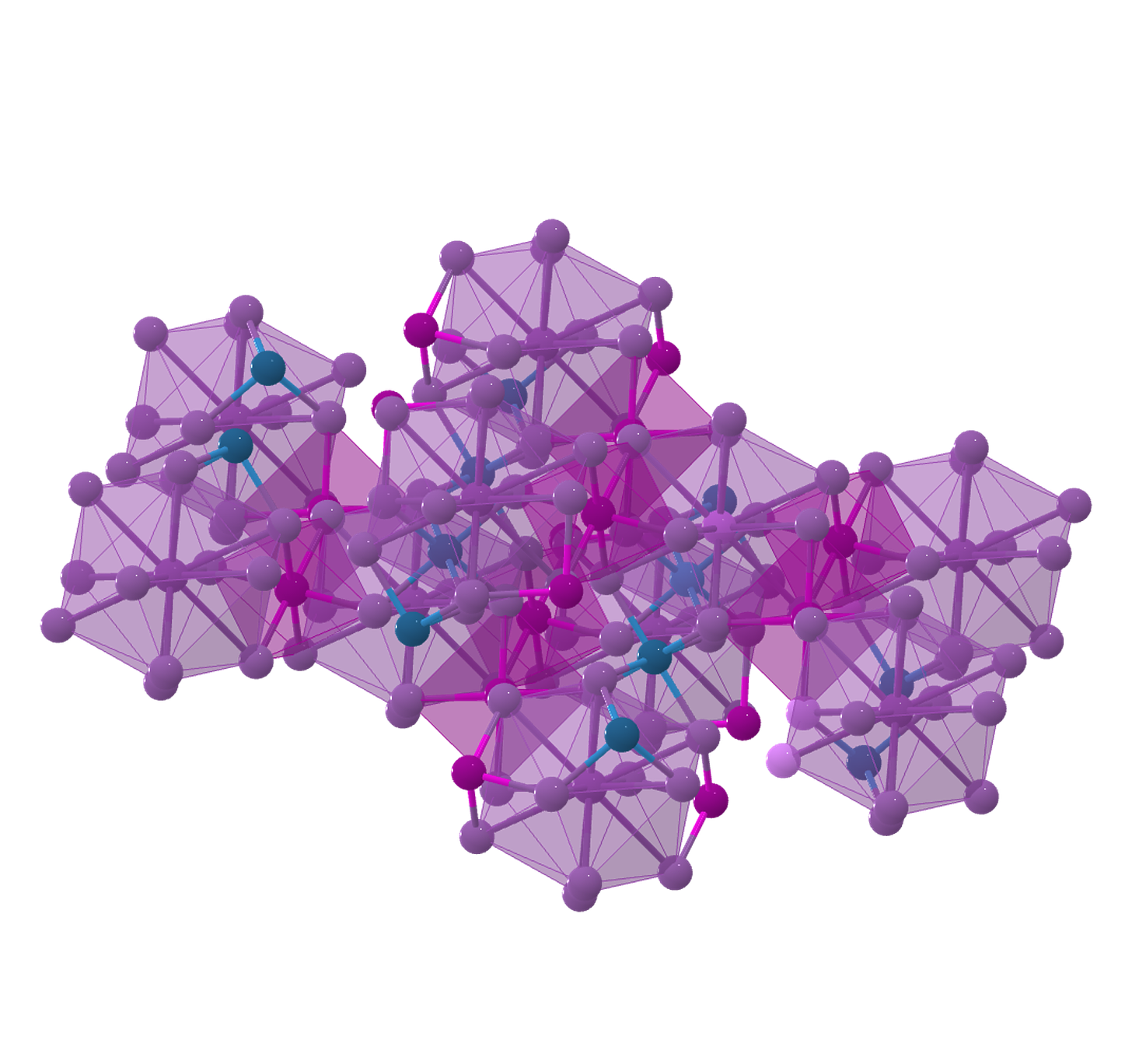
To design a material that can physically exist, you can’t simply mix a set of elements in the desired proportions. Virtually all the combinations of elements you can come up with off the top of your head aren’t “stable”, meaning that their existence is as thermodynamically impossible as unboiling an egg. (There’s surprisingly thriving research on unboiled eggs, but we’re not going to get into it here). To discover a viable material, you must either follow a synthetic route — that is, physically attempt to make it in the lab and “see”, with a set of expensive and complicated instruments, what has come out of it — or follow a computational route, evaluating each material’s stability with complex and time-consuming calculations.
AI offers a third alternative to these two approaches. After being trained on structural information about already existing materials, from common quartz to the most advanced synthetic crystals, it learns to suggest structures that are likely to be stable enough in the real world. Significantly, it does so without rigorously calculating each material’s stability (this is only done in a subsequent step), much like an experienced chemist can often tell intuitively whether a certain material or molecule is likely to exist or not. Only much, much faster.
I realized I didn’t have a word to describe how deeply materials are becoming entwined with computational processes. Technologies such as GNoME seem to challenge both the notion of the digital as some disembodied cloud separated from material bodies and the view of materiality as localized, fixed, and independent from images and language. The word metamateriality, defined as the process where linguistic models, such as AI, co-evolve with materials in symbiotic wholes, seems a good match for this emerging phenomenon.
Medianatures
The history of modern chemistry is as much a history of experimental practices as it is a history of models: representations that help us understand, and act upon, empirical realities. The fact that chemistry has been dealing with multi-scalar, unpredictable, and largely invisible phenomena for centuries has made it an incredibly fertile breeding ground for models: from the vilified “phlogiston theory” of the seventeenth century to the “molecular orbital theory” taught in schools today. What a molecule is, and that “a molecule” even exists, by the way, should not be treated as an epistemological given. The reality of molecules as we know them has been violently debated until the early twentieth century; today, one of the most successful models used in contemporary chemistry, DFT, overlooks atoms and molecules as pre-established entities to treat materials as three-dimensional clouds of probability.
This is not to say that models are interchangeable or neutral: on the contrary, by changing the models we adopt in the face of the unpredictable mutability of materials, our capacity to interact with them technologically also changes. GNoME’s enterprise further demonstrates how the work of representing, imaging, and imagining reality is never fully separable from its concrete, flesh-and-bone (or atom-and-molecule) materialization. The concept of medianatures, which I’m borrowing from Jussi Parikka’s book A Geology of Media, perfectly captures this idea: to quote the author, “[i]t is a concept that crystallizes the “double bind” of media and nature as co-constituting spheres”.
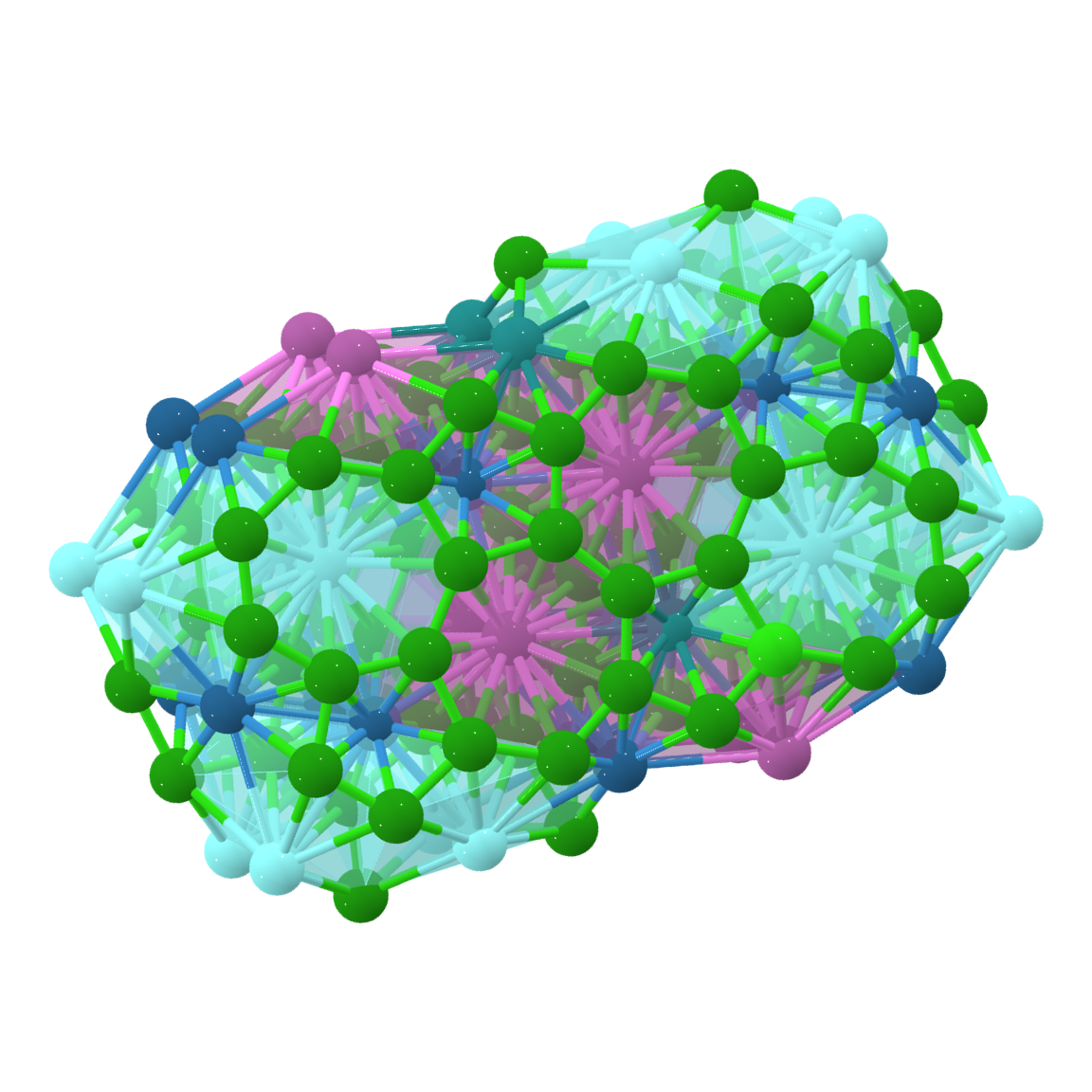
Parikka also argues that we should not fall into the trap of thinking of medianatures as futuristic, AI-driven fantasies: the entanglement of materials and models was as true at the time of Antoine Lavoisier as it is today. As AI prefigures the next generation of Earth’s minerals, however, media is becoming geological in the most literal sense. And, as much as the materiality of these bodies can be infinitely reconfigured within the abstraction of a digital mind, Earth’s crust remains (for now) the only substrate that can close the medianatural loop. Where will the exotic elements that compose DeepMind’s imagined materials eventually be mined, should we ever set out to use them? At what geopolitical, ecological, and human cost?
Mineral Evolution in the Technosphere
Geologist Robert Hazen is famous for his theory of mineral evolution: the radical idea that geological bodies, exactly like living organisms, can adapt and differentiate in response to environmental cues. Naturally, mineral evolution takes place throughout the Universe at timescales that are even more unfathomable than those of the Darwinian evolution we are familiar with; because of this, the phenomenon is even harder to notice from our human perspective. Yet, according to Hazen, there seems to be a tendency of both living and non-living matter towards developing higher complexity.
What is especially interesting about Hazen’s theory is the relationship of mineral forms with life. As I have argued elsewhere, this relationship is often symbiotic: not only did life on Earth emerge thanks to the presence of minerals, but new minerals also evolved due to planetary transformations engendered by biological life. The Great Oxidation Event, during which cyanobacteria “terraformed” our planet by releasing huge amounts of oxygen into Earth’s atmosphere, also resulted in an explosion of unprecedented geochemical structures. We don’t know if these biogenic minerals could emerge in other conditions, given enough time; but there is no doubt that life, by changing the atmosphere of the planet it inhabits, “artificially” accelerates mineral evolution processes by orders of magnitude.
Benjamin Bratton proposes a fascinating perspective on the concept of artificiality in his 2019 essay The Terraforming. Instead of thinking of the artificial as opposed to the natural, Bratton argues that we should begin to understand chemistry, geology, and biology as permeated by artificial processes which are themselves the expression of an extended planetary sentience. Thus, technology does not exist on an ontologically separate layer from what we have come to define as “nature”; instead, “technology emerges in ecological and geological context — as a folding of the planet into particular forms”.
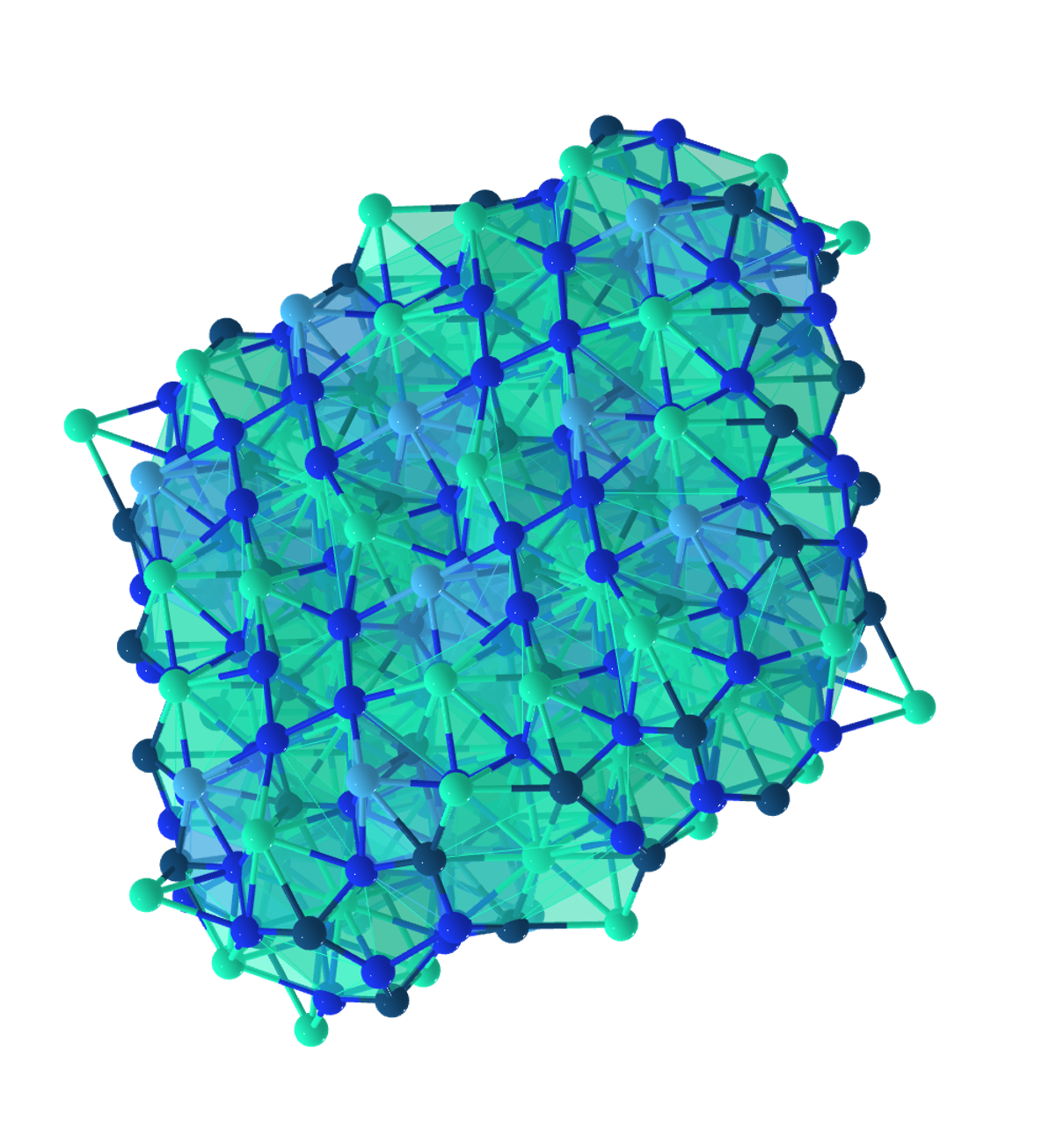
Among other things, reading The Terraforming made me reevaluate Vladimir Ivanovič Vernadsky’s well-known model of our planet as a set of “spheres” that sit one on top of the other: the geosphere, with its inert layer of rocks and minerals; the biosphere, with its proliferation of living organisms; and, finally, the noosphere, emerging as a result of the evolution of human consciousness. Although these separate “spheres” interact with, and determine the emergence of, one another, Vernadsky’s vision still betrays a belief in the primacy of intelligence as a purely human attribute. What happens if, instead of separating geology, biology, and language into discrete spheres, we begin to understand our planet as one integrated, always already artificial layer — the technosphere?
Dreams of Silicon
The GNoME project is endearing precisely because it subverts expected hierarchies between the natural and the artificial: while we are familiar with the idea of technologies emerging from geological resources, we rarely understand technology as capable of generating new geologies. If we adopt the view that inorganic structures, too, are involved in processes of selection and differentiation, then the discovery of 2.2 million new forms of previously unknown materials has the potential of setting off an exponential acceleration of mineral evolution on our planet. Who (or what) is driving this evolution? Is it simply a result of human willpower, or is it something else?
Regardless of whether we choose to apply the (widely contested) anthropomorphic vocabulary of “thinking” and “dreaming” when speaking of nonhuman technologies, there is something enchanting, and possibly even ominous, about the realization that engineered chips of doped silicon are beginning to visualize and materialize imaginary crystals. One day, some of these same crystals might become the core of the computational machines of the future; what materials will these technologies dream of, next?
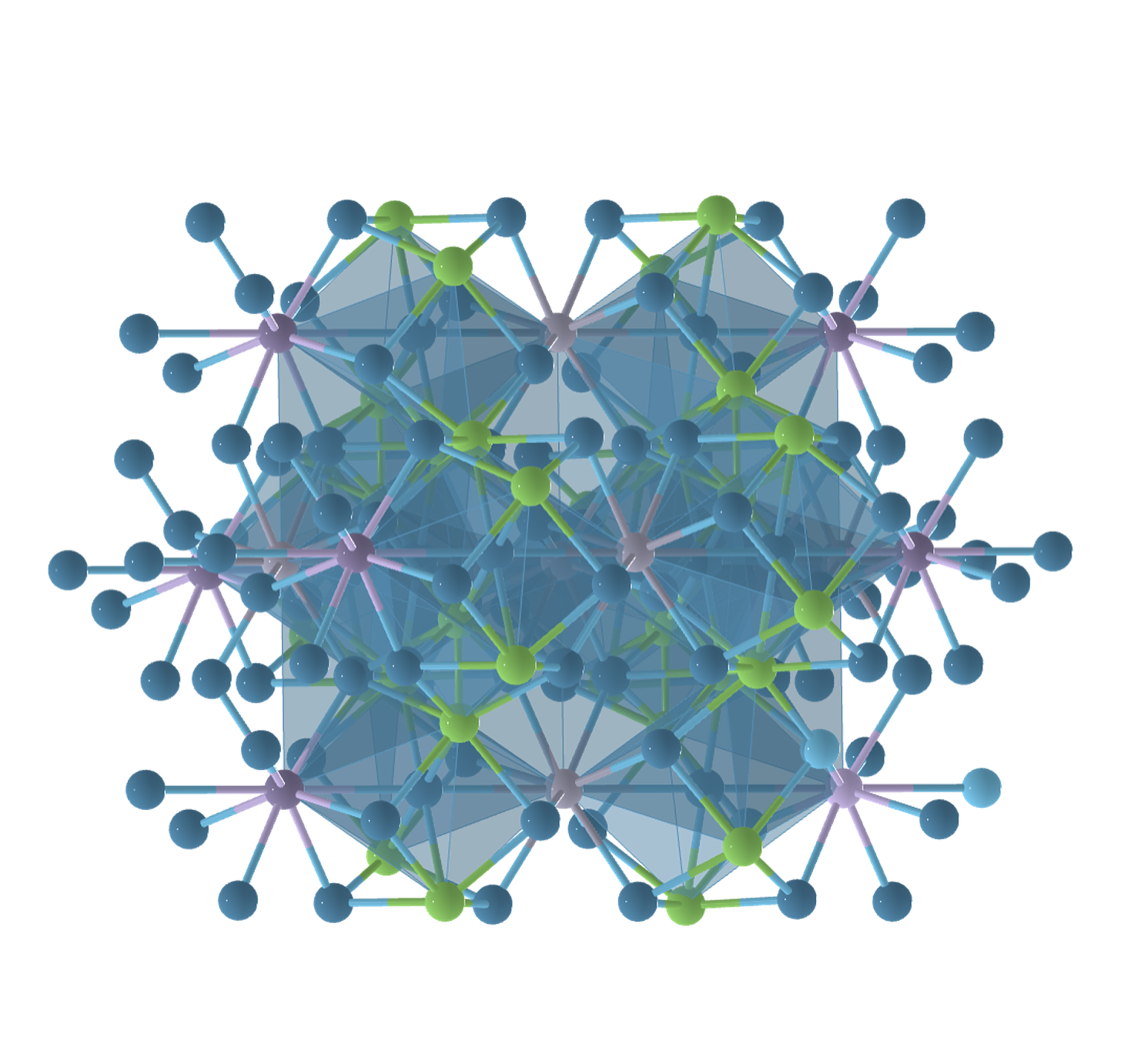
We should not be too quick to sound the trumpets of “the singularity”. One of the questions that remains open is how easily these AI-generated materials will translate into real-world substances; and while some of them were already successfully synthesized (by none other than a robotic scientist in a fully automated lab), there is still a long way to go before all traditional, human-powered chemistry is dismantled — if it ever happens. More speculatively, I think it’s interesting to ask whether our AI-centered narratives of technological convergence aren’t missing something; if they aren’t simply repeating the same old story about how nothing, not even the crudest forms of matter, can escape representational language.
Clearly, the notion of technology as a cultural tool that serves human agency has already reached its expiration date. The growing entanglements of computational processes and material bodies are making it increasingly obvious that — to quote a recurring phrase in Frank Herbert’s Dune — all technologies are animated by their own “terrible purpose”. We, however, should remain careful not to mistake this purpose for a universal master plan.
References
Merchant, A., Batzner, S., Schoenholz, S.S. et al. Scaling deep learning for materials discovery. Nature 624, 80–85 (2023).
Jussi Parikka, A Geology of Media, University of Minnesota Press, Minneapolis, 2015.
Benjamin H. Bratton, The Terraforming, Strelka Press, Moscow, 2019.
I want to thank Benjamin Bratton and Michael Nanopoulos for recommending this topic to me.
This is truly amazing, and this "One day, some of these same crystals might become the core of the computational machines of the future; what materials will these technologies dream of, next?" is poetry